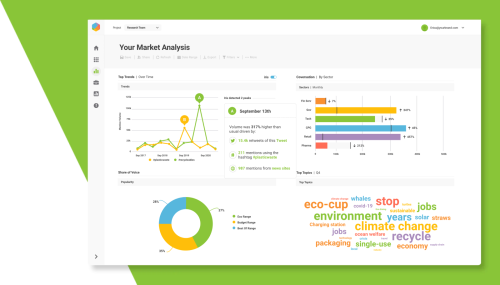
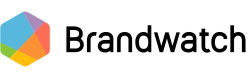
Brandwatch
Applying research & design processes to a SaaS data science team and their AI models
Senior product designer and researcher
Permanent (2021-present)
Background
Brandwatch is a leading social analytics platform that allows businesses and their analysts to gain insights from consumer’s social media activity. These insights are used by (typically global, enterprise) businesses to help plan and measure their marketing strategies and product innovations. It provides services to clients such as Meta, Google, Bank of America, GSK, Unilever and Disney.
Problem
I joined Brandwatch’s AI team who focus on augmenting raw data into insights so that expert analysts can work faster and non-experts can gain value with less skills. Designing and delivering data science AI and ML features previously was a monolithic effort and I found that engineering teams were often embarking without research and spending months siloed without any user feedback. It wasn’t uncommon for long projects to fail or for unfit features to be released. These wasted efforts meant that competitors were moving ahead and our clients were inevitably churning making work very difficult for our sales and CSM teams.
Who I worked with
C-suite
SVP & VP product, research, engineering & data science
PhD level data scientists & engineers
Product directors & owners
Lead researchers & UI designers
Customer support & sales teams
Solutions consultants & product marketers
Solution
Having worked using Lean and user-centred design processes for years, my goal was to apply these to our team so that we had confidence that we were using our valuable technical efforts in the right direction. We needed to get a better understanding of our users and show them a variety of solutions before focusing on one. Key activities to achieve this were:
Generative research and discovery phases to inform strategy
Team and stakeholder ideation sessions to create and test multiple concepts
Prototyping with real data to gain accurate user feedback
This mindset change took several months of internal conversations and relationship building to establish. This required earning the trust of several directors and a team of 40 engineers and data scientists as well as the promise to guide and support them in this new process.
Outcome
The team have
- Stopped working in development or data science silos without user or team feedback
- Scoped technical explorations so that they are time-capped and goal oriented
Understood that a variety of solutions must be explored before committing… and that design and research can support this via concept testing
Understood that we need to fail fast, and use quickly built, data driven prototypes to achieve this
Attended numerous research sessions, whether generative (understanding user needs/pains) or evaluative (seeing their prototypes being used)
“Ben's skillset is unique: he functions equally well as a senior UX researcher and a senior product designer, and that means he can shepherd a project from the early discovery / problem definition phases all the way through delivery and evaluation. I worked with him in my capacity as product director for a team of about 35 engineers and data scientists building AI-oriented SaaS products, and I relied on him not only as an individual contributor for specific projects, but also as a leader who introduced and improved product development process for the entire team…
…He has a better understanding of the user experience side of AI products than anyone else that I've worked with”
Paul Siegel - Product Director
Key activities / projects
Rapid prototyping with data science teams and their models
Getting feedback on analytics and research products relies almost completely on a user seeing their own data in an app. Traditional flat concepts only go so far in garnering useful information. Therefore we realised very quickly that we needed to prototype with data, however achieving this in the platform was a slow process that didn’t lend to innovating. Our solution came in the form of a data science 'sandbox' where we could code quickly, away from the production codebase, whilst using client data. After ideating in workshops we prototyped concepts in this sandbox and tested with users. This sped the process up by months and shifted the workload away from over burdened engineers and towards our less constrained data scientists.
Trend detection and analysis feature
AI and ML features help speed up user's time-to-insight and are powerful features for sales teams. Our data science team created an algorithm that identified shifts in activity in certain topics or communities and presented these to users so that they could spot opportunities to market and develop around these trends. Being first-to-market around a trend is a key advantage.
After researching to establish user needs, I worked with a designer to run ideation workshops with a tribe of 40+ people who created concepts for the algorithm. We undertook testing of the algorithm with user data to validate it’s accuracy and plan iterations. I am currently planning and executing a closed beta research study to assess how the model needs to be refined before it is rolled out to the entire client base.
Building relationships with client facing teams
To further understand our users, in the context of the sales process and account support, I used my research skills to interview our client facing staff. For me, understanding the business side of a project is critical in ensuring that the feature gains traction; whether that's challenging competitors or enabling renewals.
Quick delivery of critical features
Sometimes a thorough and lengthy process isn't appropriate. Product managers and directors have spent time with customers, who have upvoted for roadmap features and we are clear on what needs delivering. Working quickly with PMs and engineers we brought those features to life and released them (Using a Figma design system made the job even quicker).
To seek user feedback we guerrilla tested with staff who use our products, and the users who requested the feature. Both were quick and easy: we hopped on informal calls then noted key comments. A process which took less than a week.
"Ben worked closely with me on the Brandwatch Engagement Score - a feature that delivered 8 weeks of continuous user growth and became the fastest adopted metric. This impacted ~$700k MRR receiving the highest adoption from our Enterprise user base."
Adam Brons-Smith - Senior Product Manager
Lessons learned
- Take time to understand the whole business. Go beyond users and stakeholders and interview client facing teams which will help you fill in the blanks about why a project may be so important, and what it needs to succeed.
- Data scientists appear to be engineers however they actually work far further upstream in the realm of new concepts. They are more like designers and likely require a process and mindset from that role.
- To change a large teams process you need to listen to that team, earn their trust and then be responsible for supporting their change.
- When concepting, get architectural and system engineers to review designs for technical feasibility and remove the chance of a project hitting those barriers later in implementation.